

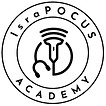
Lung Ultrasound Online Self-Training vs. Faculty Training for Medical Students
Background: Changes in medicine over the last two decades have led to more practical approaches in evaluating patients in the acute setting. Specifically, point-of-care ultrasound (PoCUS) is being used more frequently, and has become integrated into the physical examination as an essential component of patient evaluation 1-3. Advantages of PoCUS include feasibility, accuracy, and efficiency, allowing a reduction in time to diagnosis with minimal potential adverse effects 2,4.The International Federation of Emergency Medicine (IFEM) and the American College of Emergency Physicians (ACEP) endorse the use of PoCUS in acute care, citing it as an essential skill that should be learned in early residency training 23,24. PoCUS is particularly useful for quickly diagnosing lung pathologies, such as pulmonary edema, lung consolidation in pneumonia, pneumothorax, and pulmonary effusion, and as a result, helps guide subsequent care 14. PoCUS may be more accurate than the physical exam in diagnosing these pathologies, however, an important limitation is that the technology must be in the hands of a skilled operator 5–10.
Objective: The feasibility of utilizing an E-learning platform for lung PoCUS has not been explored. Therefore, in this prospective, single-blinded interventional trial we sought to compare three groups of medical students in order to determine if using an online, self-learning, lung PoCUS module is non-inferior to traditional bedside PoCUS training in examination skills and diagnosing lung pathologies. Additionally, we wanted to determine whether these learning methods were superior to no prior lung PoCUS training.
Methods: The subjects in the study were divided into three groups:
-
The intervention group: 16 students, all 3rd-year graduates, who have learned lung ultrasound using the online module solely.
-
First control group: 8 medical students, 3rd-year graduates, without previous lung ultrasound knowledge.
-
Second control group: 27 medical students, on their 3rd year of Medical School of International Health (MSIH), who have learned lung ultrasound as part of their medical school curriculum, using bedside teaching.
The intervention group was granted access to a lung ultrasound online training (eMedical Academy). Then, all three study groups were tested on several lung pathologies, using a two-part exam: Pathology recognition – on the Simbionix simulator. Three random pathologies were presented, and the students were asked to name the presented pathology for a total score of 3. Pathology recognition – from real lung ultrasound deidentified pathological clips. The students received a 10-slides long presentation and were asked to name the presented pathology, for a total score of 10. All pathologies were taught in the schools’ formal curriculum. Finally, the examiner gave a score, ranging from 0-5, on handling the probe and performing the ultrasound examination. The maximum score in the exam was a total score of 18 points.
Results: The intervention group had a significantly higher score in the total test (13.2±3.7, p.v<0.001) compared to the untrained group, and a higher score compared to the regular curriculum group (11.15±2.7, p.v = 0.097). The intervention group had a significantly higher score (p.v=0.03) in handling (4.27±1.3) compared to the regular curriculum group (3.67±1.07).
Conclusion: An online, self-learning, lung PoCUS module is non-inferior to traditional bedside PoCUS training in examination skills and diagnosing lung pathologies.
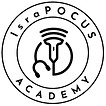
Abstract: The Effect of Real Time quality indicator Tool on Cardiac Ultrasound Performance Among Medical Students
Introduction: Point of care ultrasound (POCUS) has received in recent years a growing amount of attention as a central part of the modern physical exam 1. Its value in clinical decision making when used by internal doctors, has been established 2-4. As an operator dependent modality, it has become clear that there is a need to develop efficient training tools for large numbers of medical doctors and students to learn the proper use of the POCUS 5. One such tool is incorporation of POCUS training in medical school curriculum, and early training. The already-full curriculum and the vast resources required for this training make it essential to find effective tools to support it 6. Besides depending on proficiency that can be acquired with time and experience, some POCUS manufacturers have also added user-interface functions to their devices which hold the potential to help enhance abilities of novice and experienced users alike. One such feature is the Real-Time quality indicator, integrated in the Ejection Fraction (RTEF) tool which executes automated calculations of ejection fraction values in apical 4 chamber (A4CH) position. This quality indicator provides the user with real-time feedback on the quality of the A4CH image produced. The quality indicator tool presents the user with a contour line in green, yellow, or red, based on an AI algorithm which analyzes image quality, A4CH view identification, and EF results consistency. According to data we have collected over the last five years, among the views commonly used in echocardiography, preliminary study results show the apical views are the most challenging views to achieve. We believe the quality indicator can help during the learning process by giving real time feedback during image acquisition.
Methods: In our research we explore the use of the quality indicator tool as a learning aid and for live assistance in image acquisition and test its potential as both a teaching device and an enhancer of the POCUS handling skills. We have conducted a medical education focused prospective, single blinded randomized controlled trial. The two-month study was integrated in the pre-clinical clerkship course of 4th year medical students. 100 students participated in the study and underwent an 8 -hour cardiac ultrasound training course, as part of their curriculum. The students were divided in two groups- an intervention group (N=27) using the quality indicator tool for the apical 4 chamber view, and a control group (N=73) using the standard POCUS device with no quality indicator. In our study we compare the two group’s exam scores for apical 4 chamber view, in addition to particularly examining image quality and time to image acquisition.
Results: We are currently in the process of data interpretation and pending results, which will be presented by time of presentation.
Bibliography
1. Narula J, Chandrashekhar Y, Braunwald E. Time to add a fifth pillar to bedside physical examination: Inspection, palpation, percussion, auscultation, and insonation. JAMA cardiology. 2018;3(4):346-350. http://dx.doi.org/10.1001/jamacardio.2018.0001. doi: 10.1001/jamacardio.2018.0001.
2. Golan YB, Sadeh R, Mizrakli Y, et al. Early point-of-care ultrasound assessment for medical patients reduces time to appropriate treatment: A pilot randomized controlled trial. Ultrasound in Medicine and Biology. 2020;46(8):1908-1915. https://www.umbjournal.org/article/S0301-5629(20)30139-3/abstract. Accessed Sep 14, 2021. doi: 10.1016/j.ultrasmedbio.2020.03.023.
3. Croft LB, Duvall WL, Goldman ME. A pilot study of the clinical impact of hand-carried cardiac ultrasound in the medical clinic. Echocardiography. 2006;23(6):439-446. https://onlinelibrary.wiley.com/doi/abs/10.1111/j.1540-8175.2006.00240.x. Accessed Sep 19, 2021. doi: 10.1111/j.1540-8175.2006.00240.x.
4. Kobal SL, Atar S, Siegel RJ. Hand-carried ultrasound improves the bedside cardiovascular examination. CHEST. 2004;126(3):693-701. https://journal.chestnet.org/article/S0012-3692(15)31207-1/abstract. Accessed Oct 15, 2021. doi: 10.1378/chest.126.3.693.
5. Gilbertson EA, Hatton ND, Ryan JJ. Point of care ultrasound: The next evolution of medical education. Annals of translational medicine. 2020;8(14):846. https://search.proquest.com/docview/2434478973. doi: 10.21037/atm.2020.04.41.
6. Kobal SL, Lior Y, Ben-Sasson A, Liel-Cohen N, Galante O, Fuchs L. The feasibility and efficacy of implementing a focused cardiac ultrasound course into a medical school curriculum. BMC medical education. 2017;17(1):94. https://www.ncbi.nlm.nih.gov/pubmed/28558692. doi: 10.1186/s12909-017-0928-x.
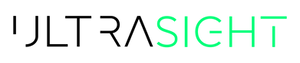
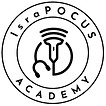
The effect of real-time AI-based software on Cardiac Ultrasound performance among Medical Students and Interns
Introduction: The use of point-of-care ultrasound (POCUS) is rapidly growing thanks to its value in improving diagnostic accuracy and shortening the time needed for appropriate treatment. As an operator-dependent modality, dedicated training in acquiring and interpreting ultrasound images is required.
In contrast to previous studies, which mainly focused on improving acquiring the POCUS competency virtually AI-operated modules, such as UltraSight's, have been created to improve efficiency while hand-on training is performed. UltraSight's application provides on-screen instructions to emulate how a sonographer would manipulate the transducer to acquire the optimal view. Furthermore, using the Quality Bar indicator, UltraSight's AI software also analyzes the cardiac ultrasound image in real-time and indicates when an image is of sufficient quality to be diagnostic.
Objective: This study aims to compare both short-term and long-term success rates in acquisition and quality of basic cardiac ultrasound views between medical students practiced using the guidance of AI-based software and students who underwent traditional hand-on cardiac ultrasound courses.
Methods: This randomized controlled study includes two groups:
-
Intervention group: 20 interns and medical students will undergo cardiac ultrasound training, assisted by the AI tool.
-
Control group: 20 interns and medical students who will undergo the same traditional cardiac ultrasound training without the assistance of the AI tool.
The one-month study will take place at Ben Gurion University. All 40 participants will undergo a 6-hour cardiac ultrasound training, followed by a 6-minute test. Twenty students, comprising the intervention group, will be trained using UltraSight's software assistance, while the rest will be using the same POCUS device without the AI software.
Results:
Conclusion:
_______________
